You signed in with another tab or window. Reload to refresh your session.You signed out in another tab or window. Reload to refresh your session.You switched accounts on another tab or window. Reload to refresh your session.Dismiss alert
Hi, I tried the pretrained laion prior and decoder weights to test the pipeline for image inpainting. However, I have been getting bad results (Blur in the inpainting area - picture attached!). I tried playing around with the cond_scale, but I had no luck.
def is_list_str(x):
if not isinstance(x, (list, tuple)):
return False
return all([type(el) == str for el in x])
one_text = isinstance(text_input, str) or (not is_list_str(text_input) and text_input.shape[0] == 1)
if isinstance(text_input, str) or is_list_str(text_input):
text_input = [text_input] if not isinstance(text_input, (list, tuple)) else text_input
text_input = tokenizer.tokenize(text_input).to('cuda')
Hi, I tried the pretrained laion prior and decoder weights to test the pipeline for image inpainting. However, I have been getting bad results (Blur in the inpainting area - picture attached!). I tried playing around with the cond_scale, but I had no luck.
Here is my implementation code:
!pip install dalle2-pytorch==1.1.0
!wget -O 'prior_weights.pth' 'https://huggingface.co/laion/DALLE2-PyTorch/resolve/main/prior/latest.pth'
!wget 'https://huggingface.co/laion/DALLE2-PyTorch/raw/main/prior/prior_config.json'
!wget -O 'decoder_weights.pth' 'https://huggingface.co/laion/DALLE2-PyTorch/resolve/main/decoder/v1.0.2/latest.pth'
!wget 'https://huggingface.co/laion/DALLE2-PyTorch/raw/main/decoder/v1.0.2/decoder_config.json'
Prior Setup
prior_config = TrainDiffusionPriorConfig.from_json_path("/content/prior_config.json").prior
prior = prior_config.create().cuda()
prior_model_state = torch.load("/content/prior_weights.pth")
prior.load_state_dict(prior_model_state, strict=True)
Decooder Setup
decoder_config = TrainDecoderConfig.from_json_path("/content/decoder_config.json").decoder
decoder = decoder_config.create().cuda()
decoder_model_state = torch.load("/content/decoder_weights.pth")['model']
for k in decoder.clip.state_dict().keys():
decoder_model_state["clip." + k] = decoder.clip.state_dict()[k]
decoder.load_state_dict(decoder_model_state, strict=True)
Run config:
text_input = ['Bowl of oranges']
mask_path = '/content/mask.png'
img_path = '/content/img.png'
def is_list_str(x):
if not isinstance(x, (list, tuple)):
return False
return all([type(el) == str for el in x])
one_text = isinstance(text_input, str) or (not is_list_str(text_input) and text_input.shape[0] == 1)
if isinstance(text_input, str) or is_list_str(text_input):
text_input = [text_input] if not isinstance(text_input, (list, tuple)) else text_input
text_input = tokenizer.tokenize(text_input).to('cuda')
text_cond = text_input
def read_image(path):
Load the image and resize it
img = Image.open(path)
img = transforms.Resize((256, 256))(img).convert("RGB")
Convert the image to a NumPy array and then to a PyTorch tensor
img_array = transforms.ToTensor()(img)
tensor = torch.reshape(img_array, (1, 3, 256, 256))
p = tensor.numpy()
return tensor
def read_mask(mask_path):
mask = Image.open(mask_path).convert('RGB').resize((256, 256), resample=Image.BICUBIC)
mask_binarized = np.array(mask.convert('1'))
mask_binarized_flipped = np.where(mask_binarized == 0, 1, 0)
cv2_imshow(mask_binarized_flipped * 255)
mask_binarized_flipped.resize(256, 256, 1)
return torch.from_numpy(mask_binarized_flipped).permute(2, 0, 1)
image_embed = prior.sample(text_input, num_samples_per_batch = 2, cond_scale = 10)
inpaint_image = read_image(img_path).cuda() # (batch, channels, height, width)
inpaint_mask = read_mask(mask_path).bool().cuda() # (batch, height, width)
inpainted_images = decoder.sample(
image_embed = image_embed,
text = text_cond,
inpaint_image = inpaint_image,
inpaint_mask = inpaint_mask
)
images = inpainted_images.cpu()
for img in images:
img = ToPILImage()(img)
img.show()
Here is the input image:
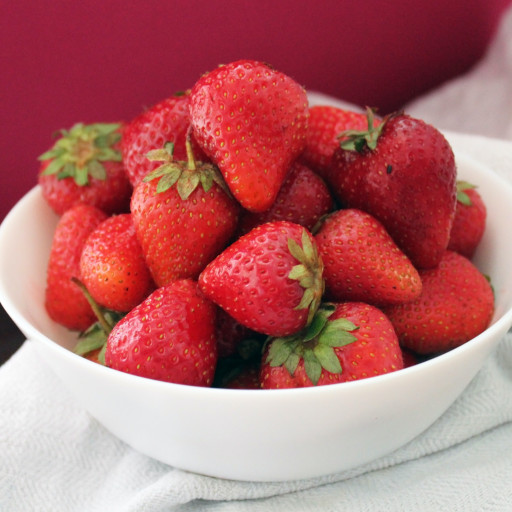
Here is the input mask:
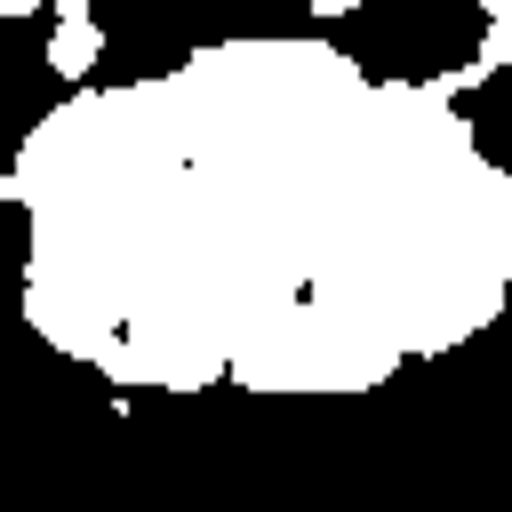
This is the result I am getting for the prompt : "Bowl of oranges"

Does anybody have any tips or could direct me to anything I may be doing wrong?
Thank you!
The text was updated successfully, but these errors were encountered: